Translational Biophotonics in Developmental Disorders and Diseases
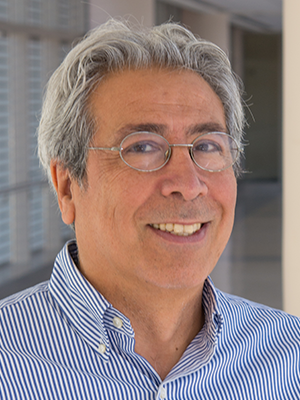
- Amir H. Gandjbakhche, PhD, Head, Section on Translational Biophotonics
- Hadis Dashtestani, PhD, Postdoctoral Fellow
- Thien Nguyen, PhD, Postdoctoral Fellow
- Jinho Park, PhD, Postdoctoral Fellow
- Soongho Park, PhD, Postdoctoral Fellow
- Wan-Chun Su, PhD, Postdoctoral Fellow
- Wei Lun Huang, MS, Intramural Research Training Award Student
- Emily Blick, BS, Postbaccalaureate Fellow
- Aaron Buckley, BS, Postbaccalaureate Fellow
- Sara Johnson, BS, Postbaccalaureate Fellow
- William Martin, BS, Postbaccalaureate Fellow
- John Millerhagen, BS, Postbaccalaureate Fellow
- Vinay Veluvolu, BS, Postbaccalaureate Fellow
- Marc Bornstein, PhD, Special Volunteer
- Han-Shin Hahn, PhD, Special Volunteer
- Kosar Khaksari, PhD, Special Volunteer
- Siamak Aram, PhD, Guest Researcher
Utilizing functional near-infrared spectroscopy (fNIRS) in conjunction with complementary neuroimaging techniques, we investigated neural activity in two distinct cohorts: (1) a group of healthy volunteers; and (2) infants and children, both with and without developmental disorders. The primary aim of the first cohort was to validate fNIRS findings through comparison with previous results obtained from functional MRI (fMRI) and EEG studies, while the focus of the second cohort was to comprehensively explore the typical and atypical developmental trajectories in infants and children, including those at elevated risk for developmental disorders.
Before conducting fNIRS studies, we engaged in an exhaustive assessment of contemporary fNIRS findings pertaining to the tracking of neurodevelopmental trajectories in infants and children, encompassing those with or without developmental disorders [Reference 1].
In relation to the adult cohort, we successfully conducted a pilot study encompassing 30 healthy volunteers, aimed at assessing the viability of fNIRS for the study of the mirror neuron network (MNN). The culmination of our endeavors revealed compelling outcomes. Specifically, our findings highlight parietal regions, including the bilateral superior parietal lobule (SPL), bilateral inferior parietal lobule (IPL), the right supra-marginal region (SMG), and the right angular gyrus (AG) as potential candidate regions within the human MNN. Furthermore, we effectively demonstrated the paradigm's capacity to discern variations in subclinical levels of autistic traits, presenting an avenue for subsequent exploration in clinical populations characterized by challenges in action comprehension and representation, notably autism spectrum disorders (ASD) [Miguel et al., PloS One 2021;16:e0253788]. Subsequent to this, a supplementary analysis utilizing a connectivity approach was undertaken [Nguyen et al., Brain Sci 2021;11:397]. Notably, our findings revealed significant interplay between various brain regions during action execution, with distinct region-to-region connections within the left hemisphere apparent while participants executed actions with their right hand. These connections included interactions within the left precentral, left postcentral, left inferior parietal regions, as well as between the left supramarginal and left angular regions.
To comprehensively delineate the MNN utilizing concurrent EEG and fNIRS signals, we developed a multimodal, multiset data-fusion analysis, strategically leveraging the strengths of each neuroimaging modality, that is, the EEG's exceptional temporal resolution and the fNIRS's superior spatial resolution. In this pursuit, we harnessed the well established Mu suppression phenomenon as an indicator of MNN activation in EEG–related neural activity. By synergistically employing EEG and fNIRS, each modality contributing its unique attributes, we achieved a more refined understanding of MNN development. Specifically, we applied a structured sparse multiset canonical correlation analysis (ssmCCA) model to our merged dataset, revealing congruent activity patterns during both action execution and observation across designated regions of interest. These results underscored heightened brain activity within the left hemisphere's paracentral, precentral, and inferior and superior parietal regions during action execution. This was the first report that fused distinct brain metrics (hemodynamic response function and electrical activity) characterize the MNN in the human brain [Dashtestani et al. Sci Rep 2022;12:6878]. Subsequent to our in-depth exploration of brain activity patterns during action execution and observation, we investigated the imagination condition in relation to both action execution and observation. The intrinsic value of introducing this dimension lies in the belief that motor cognitive abilities hinge on, and are cultivated through, the mechanisms of mental rehearsal and re-enactment of action execution. Our findings indicated the pivotal role of the left parietal inferior region as the primary contributor within the imagination condition. Concurrently, additional brain regions within the left hemisphere exhibited activation during the motor imagery task, mirroring the patterns witnessed during execution and observation conditions (Figure 1). Despite the relatively limited literature on action imagination, our outcomes harmonize with existing studies, validating the notion that both action observation and imagination stimulate the same sensory-motor cortical network that underpins the execution of the corresponding action. One plausible explanation for these consistent brain activity patterns across the three conditions is attributed to the internal rehearsal of action, reinforcing the intrinsic connection between observation, imagination, and execution [Reference 2].
Figure 1. Cross-modality correlations and associated p-values during motor execution (ME), motor observation (MO), and motor imagery (MI) (A, image at right), and color-coded regions associated with execution, observation, and imagination (B, table below).
The color bar refers to the p-values of the correlations in the region. Moderate correlations were found over the left inferior parietal lobe (IPL) (r=0.39; p=0.059), left posterior central gyrus (POCG) (r=0.38, p=0.061), and left supramarginal region (SMG) (r=0.29, p=0.069) during ME; over the left IPL (r=0.46; p=0.053), left SMG (r=0.38; p=0.059), and left POCG (r=0.32; p=0.066) during MO; and over the left SMG (r=0.50, p=0.059), left POCG (r=0.41; p=0.061), and left IPL (r=0.20, p=0.063) during MI.
Execution | Observation | Imagination | |||||||
---|---|---|---|---|---|---|---|---|---|
Correlation | fNIRS region | P-Value | Correlation | fNIRS region | P-Value | Correlation | fNIRS region | P-Value | |
Comp 1 | 0.391 | Left IPL | 0.059 | 0.455 | Left IPL | 0.053 | 0.4966 | Left SMG | 0.059 |
Comp 2 | 0.317 | Left POCG | 0.061 | 0.382 | Left SMG | 0.059 | 0.4070 | Left POCG | 0.061 |
Comp 3 | 0.294 | Left SMG | 0.069 | 0.316 | Left POCG | 0.066 | 0.2017 | Left IPL | 0.063 |
Comp 4 | 0.3350 | Left PRCG | 0.0653 | 0.3351 | Left PRCG | 0.0617 | 0.300 | Left PRCG | 0.0664 |
COVID-19 point-of-care biosensor
At the beginning of the COVID-19 pandemic, our section proposed to develop a biosensor to monitor patients with respiratory infectious diseases such as COVID-19. We designed a wearable and wireless device, which can measure body temperature, chest movement, cardiac and respiratory functions, and tissue oxygenation parameters. We then conducted a clinical protocol entitled ‘A Pilot Study to Evaluate a Noninvasive Multimodal Biosensing Device for Screening and Monitoring Response to Treatment of Infectious Respiratory Diseases’ to evaluate performance of the device in healthy subjects at rest and during induced hypercapnia, breath holding, and paced breathing. Thirty-six volunteers were enrolled in the study, and measurements were performed on 26 participants. We are currently performing data analysis on the collected data.
In parallel with research at NIH, we were collaborating with Babak Shadgan to collect data on 24 healthy volunteers during normal breathing, restricted breathing, and rapid breathing through a clinical protocol. Tissue oxygenation levels and hemodynamics responses, including oxy-, deoxy-, and total hemoglobin, were measured using an NIRS device. The device consists of a multi-wavelength LED emitting light at 760 nm and 850 nm, and three photodiode detectors 3, 3.5, and 4 cm away from the LED. The NIRS device was placed over the participant's chest during data acquisition. In order to obtain signals similar to the breathing patterns experienced by COVID-19–infected patients, three breathing patterns, i.e., normal breathing, restricted breathing, and rapid breathing, were defined. In our section, two deep-learning classification algorithms for breathing patterns were developed based on convolutional neural network (CNN). The first algorithm is a classification model consisting of 113 layers. The residual concept was applied to prevent the vanishing gradient problem that occurs when the depth of the deep-learning model increases. 64-length (corresponding to 6.4 seconds) oxy-hemoglobin data signals were used to learn the classification model. We achieved a classification accuracy of 91.77% using this model [Reference 3].
The second model is an evolved version of the first model. The model improves classification accuracy by using additional compressed features that can form the data. We added an auto encoder model to generate compressed features of the data. The features compressed in the auto-encoder were fused with the features generated by the first classification model (Figure 2). This model was trained on the 64-length oxy-hemoglobin data signals. We achieved an accuracy of 92.34% using this model.
Figure 2. Architecture of deep learning model to classify different breathing patterns
Architecture of breathing pattern classification model with auto-encoder: The developed model consists of an auto-encoder network for generating compressed features and a classification network for data class classification. The auto encoder's encoder network is trained to compress data, and the decoder network is trained to reconstruct the input signal. The embedded features generated from the encoder are combined with the features generated from the classification network and then used to classify the classes.
Placenta oxygenation: from basics to point of care
Placental insufficiency is a condition in which the placenta fails to provide adequate nutrients or oxygen to the fetus, a condition that is associated with pregnancy complications such as fetal growth restriction, fetal death, preterm labor, and other complications. However, there is no available technology to assess placental condition. Our section proposed the use of NIRS to measure the placental oxygenation level, and then to utilize this parameter to evaluate the condition of the placenta. NIRS is a noninvasive optical technique to measure tissue hemodynamics, including oxy- and deoxy-hemoglobin concentration and tissue oxygenation. In a typical NIRS device, there is a light source, which shines NIR light into tissue, and a light detector, which detects reflected light. Based on the distinct absorption spectrum of oxy-hemoglobin and deoxy-hemoglobin in the NIR range, we can derive concentration of these two parameters, and hence tissue oxygenation. A major problem in the assessment of placental oxygenation using NIRS arises from the anatomical location of the placenta, which lies below skin, adipose tissue, and the uterine wall. Considering these tissues above the placenta, we designed several models of a wearable depth-resolving NIRS device, featuring six source-detector distances in the range of 10–60 mm. Distinct source and detector distances scan different tissue depths to help distinguish between placental and maternal oxygenation. Our first model includes light sources with two wavelengths of 760 nm and 840 nm, which are sensitive to changes in blood oxy-hemoglobin and deoxy-hemoglobin. Using this model, under the protocol #090717MP4E, which was approved by the Wayne State University Human Investigations Committee Institutional Review Board (IRB), tissue oxygenation was measured in the placenta of 12 singleton pregnant women in their third trimester at the Center for Advanced Obstetrical Care and Research of the Perinatology Research Branch, located at the Detroit Medical Center [Nguyen et al., Biomed Optic Exp 2021;12:4119]. Five of these women had maternal pregnancy complications. Our preliminary results indicated a significantly lower tissue oxygen saturation level in the placenta of patients with complicated pregnancies (69.4% ± 6.7%) than in the placenta of their peers with normal pregnancies (75.0% ± 5.8%). After delivery, 10 of the 12 participants’ placentas were delivered to the pathology department at the Detroit Medical Center to inspect for lesions. Five placentas were found to have chronic or acute lesions, four of which belonged to participants with maternal pregnancy complications. We further found that patients with lesion-free placentas presented a significantly higher placental oxygen saturation (74.2% ± 5.8%) than patients with lesions (68.7% ± 5.6%). The results suggest a relationship between the placental oxygen saturation and pregnancy complications and placental pathology. However, with our first model, we need to use data collected from two distances (e.g., 30 and 40 mm distances) to calculate placental oxygenation level. As a result, calculated placental oxygenation level was transabdominal.
In order to target different tissue depths, we developed a method called ‘single source-detector separation’ (SSDS), which uses one distance with three wavelengths to calculate tissue oxygenation level (StO2) [Reference 4]. The method was tested using data from Monte-Carlo simulation with an average accuracy of 97%. We then performed StO2 measurements on seven healthy volunteers in the prefrontal cortex during a simulated hypercapnia test using a continuous wave (CW) NIRS device. The device consists of a light source and two photodetectors, which are 30 mm and 40 mm away from the light source. The cerebral oxygen saturation was calculated using both a conventional spatially resolved spectroscopy (SRS) approach, which uses the reflected intensities at both separations, and the SSDS approach, which employs the reflected intensities at either 30 mm or 40 mm separation. The SRS–based StO2 calculation was similar to the value calculated from the SSDS method (average difference = 5.0% ± 1.1%). We submitted a provisional patent application for the SSDS method (E-037-2023-0-US-01).
In addition, we upgraded our first model wearable device so that light sources include three wavelengths: 735, 810, and 850 nm. The second model has a data acquisition rate of 20 Hz, which allows collection of high frequency signals such as maternal and fetal heart rates. An accelerometer was added to the second model to detect fetal movement. A provisional patent application was submitted for the second model (E-198-2022-0-US-01) (Figure 3). This model has been deployed to perform a one-time measurement of 24 pregnant women at their second and third trimesters at the Detroit Medical Center. Ten of the women in this cohort had maternal complications, including chronic hypertension, asthma, type II diabetes, renal failure with dialysis, and prolactinoma, and five had preeclampsia with severe features. The experimental procedure was performed in the same way as the previous measurement, where the participant lay down on the examination bed in a supine position. After delivery, the placentas of 22 participants were sent to a pathology laboratory to examine for lesions. Seventeen placentas had issues such as acute and/or chronic inflammatory lesions, acute funisitis and vasculitis, placental infarct, and lesions associated with maternal vascular malperfusion. In general, all 24 participants had either maternal complications, placental issues, and/or neonatal complications. The average oxygen saturation of the placenta of all participants was 68.9 ± 4.2%, which was similar to the oxygen saturation level found in the group of pregnant women with maternal complications and/or placental lesions in the first measurement.
Figure 3. Data acquisition and data processing method to measure placental oxygenation level
Probing different tissue layers is critical to distinguish between maternal and placental oxygenation. Monte-Carlo methods are used to create a look-up table for this purpose.
We are in the process of designing an analysis algorithm that assesses the behavior of placental cells while taking into account different oxygen levels. The analysis is conducted in conjunction with the dynamic full-field optical coherence tomography (DFFOCT) system, simultaneously measuring placental oxygenation using the NIRS device. We are developing an algorithm designed to analyze changes in the dynamic activity (frequency and magnitude) of cellular organelle and derive a weighted mean frequency as a representative value. In our preliminary experiments, we evaluated cell viability using HeLa cells, a commonly employed immortalized human cell line in cell research. The approach we introduced is a label-free, non-invasive observation technique that permits precise quantitative analysis of cell dynamic activity without any impact on the cells themselves. Using this method, we effectively differentiated shifts in the survival status of HeLa cells by using parameters that characterize the cells' dynamic activity, with a focus on mean frequency [Park et al. Biomed Optic Exp 2021;112:6431]. Additionally, data obtained through DFFOCT is not biased by algorithmic models and has been successfully used in a variety of machine learning models, enabling highly accurate cell viability determinations. Based on our findings, we believe that DFFOCT can serve as a valuable tool for assessing variations in the dynamic activities of placental cells in relation to oxygen saturation. We hypothesize that there is a relationship between the dynamic activity of placental cells and potential neurodevelopmental disorders. In our more recent research, we explored the dynamic activity of HeLa cells by subjecting them to varying oxygen concentrations within the range of 1% to 20%, while keeping other growth conditions constant, such as temperature and a 5% CO2 environment (Figure 4). Our results reveal that the dynamic cellular movements observed at oxygen concentrations of 1% and 20% exhibit a significant difference of over 84%. We are currently analyzing the patterns of changes across different oxygen concentrations. In our future research, we plan to conduct a comparative analysis of dynamic activities in placental cells with a focus on oxygen levels, building upon the insights gained from our observations in HeLa cells (Figure 4).
Figure 4. DFFOCT for cell viability assay
Assessment of cell viability state over by trained Logistic Regression model. After (a) 0-hour 15-minute, (b) 2-hour, (c) 4-hour, and (d) 6-hour time without CO2 and maintained at 27oC. Green and red labels indicate live and dead cells, respectively. The inset white scale bar represents 100 mm.
Additional Funding
- Bench to Bedside Award 345 (2016): “Mirror neuron network dysfunction as an early biomarker of neurodevelopment” (ongoing)
- Human Placenta Project—NICHD (2016, ongoing)
- Scientific Director's Award
Publications
- Su WC, Colacot R, Ahmed N, Nguyen T, George T, Gandjbakhche A. The use of functional near-infrared spectroscopy in tracking neurodevelopmental trajectories in infants and children with or without developmental disorders: a systematic review. Front Psychiatry 2023 14:121000.
- Su WC, Dashtestani H, Miguel HO, Condy E, Buckley A, Park S, Perreault JB, Nguyen T, Zeytinoglu S, Millerhagen J, Fox N, Gandjbakhche A. Simultaneous multimodal fNIRS-EEG recordings reveal new insights in neural activity during motor execution, observation, and imagery. Sci Rep 2023 13:5151.
- Park J, Mah AJ, Nguyen T, Park S, Ghazi Zadeh L, Shadgan B, Gandjbakhche AH. Modification of a conventional deep learning model to classify simulated breathing patterns: a step toward real-time monitoring of patients with respiratory infectious diseases. Sensors 2023 23:5592.
- Nguyen T, Park S, Hill B, Gandjbakhche A. Single source-detector separation approach to calculate tissue oxygen saturation using continuous wave near-infrared spectroscopy. IEEE Open J Engineering Med Biol 2023 99:1–7.
- Park S, Veluvolu V, Martin WS, Nguyen T, Park J, Sackett DL, Boccara C, Gandjbakhche A. Label-free, non-invasive, and repeatable cell viability bioassay using dynamic full-field optical coherence microscopy and supervised machine learning. Biomed Optics Express 2022 13:3187–3194.
Collaborators
- Franck Amyot, PhD, Center for Neuroscience and Regenerative Medicine, Uniformed Services University of the Health Sciences (USUHS), Bethesda, MD
- Mehran Armand, PhD, The Johns Hopkins University, Baltimore, MD
- Claude Boccara, PhD, École Supérieure de Physique et de Chimie Industrielles, Paris, France
- Nathan Fox, PhD, University of Maryland, College Park, MD
- Andrea Gropman, MD, Children's National Health System, Washington, DC
- Jay Knutson, PhD, Laboratory of Molecular Biophysics, NHLBI, Bethesda, MD
- Guoyan Luo, MD, PhD, Howard University Hospital, Washington, DC
- Tom Pohida, MS, Division of Computational Bioscience, CIT, NIH, Bethesda, MD
- Randall Pursley, MSc, Signal Processing and Instrumentation Section, CIT, NIH, Bethesda, MD
- Jessica C. Ramella-Roman, PhD, Florida International University, Miami, FL
- Roberto Romero-Galue, MD, D(Med)Sci, Perinatology Research Branch, NICHD, Detroit, MI
- Dan Sackett, PhD, Division of Basic and Translational Biophysics, NICHD, Bethesda, MD
- Babak Shadgan, MD, MSc, PhD, University of British Columbia, Vancouver, Canada
- Audrey Thurm, PhD, Pediatrics & Developmental Neuropsychiatry Branch, NIMH, Bethesda, MD
- Bruce Tromberg, PhD, Section on Biomedical Optics, NICHD, Bethesda, MD
- Eric Wassermann, MD, Cognitive Neuroscience Section, NINDS, Bethesda, MD
Contact
For more information, email gandjbaa@mail.nih.gov or visit https://www.nichd.nih.gov/research/atNICHD/Investigators/gandjbakhche.