The Biological Impact and Function of Transposable Elements
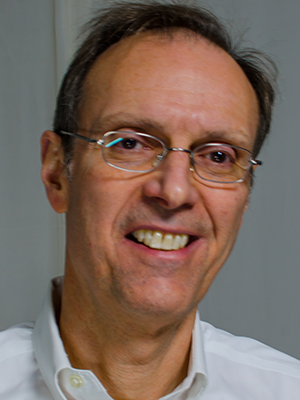
- Henry L. Levin, PhD, Head, Section on Eukaryotic Transposable Elements
- Angela Atwood-Moore, BA, Senior Research Assistant
- Caroline Esnault, PhD, Visiting Fellow
- Sudhir Rai, PhD, Visiting Fellow
- Parmit Singh, PhD, Visiting Fellow
- Anthony J. Hickey, PhD, Postdoctoral Fellow
- Si Young Lee, PhD, Postdoctoral Fellow
- Zoe Lautz, BA, Postbaccalaureate fellow
- Maya Sangesland, BA, Postbaccalaureate Fellow
- Larissa Ault, Summer Student
- Zainab Sherani, Summer Student
Inherently mutagenic, the integration of retroviral and retrotransposon DNA is responsible for many pathologies, including malignancy. Given that some chromosomal regions are virtually gene free while others encode genes essential for cellular processes, the position of integration has great significance. Recent studies showed clearly that integration occurs into specific types of sequences and that the targeting patterns vary depending on the retrovirus or retrotransposon. Currently, there is great interest in such patterns, in part because understanding the mechanisms that position HIV-1 insertions may lead to new antiviral therapies. In addition, retrovirus-based vectors are now being used for gene therapy. Early gene therapy vectors had patterns of integration that activated oncogenes and caused leukemia in patients. Therefore, to gauge the risks associated with new gene therapy vectors, it is essential that we characterize in detail the positions of integration and understand the mechanisms that position such integration.
Ultra-high throughput sequencing of transposon integration with serial number technology provides a saturated profile of target activity in Schizosaccharomyces pombe.
Tf1 integration is directed to the promoters of RNA pol II–transcribed genes and such integration can increase transcription. This raises important questions about the impact of integration, namely, what distinguishes the preferred promoters from those with less integration. Previously, we addressed these questions by isolating cells with integration of Tf1-neo and sequencing 73,000 integration sites using ligation-mediated PCR (Guo and Levin, Genome Res 2010;20:239-248). Over 90% of integration occurred within intergenic sequences that contained promoters, and most of the integration occurred in just 30% of promoters. This high level of variation was not the result of selection because the same pattern was obtained in diploid cells. Genes regulated by environmental stress are enriched in the preferred targets of integration. However, to understand factors that promote integration bias and to precisely define the integration pattern, we needed to overcome a serious limitation of the methods used to deep-sequence integration. High-throughput sequence runs of integration libraries produce hundreds of millions of sequence reads with large numbers of duplicates. The duplicate reads are typically the result of PCR amplification but could also arise from multiple integration events at the same chromosomal nucleotide. No method existed to distinguish between PCR bias and independent integration, so we and others published only the position of integration, not the number of independent events. The approach was reasonable until integration data sets became so large that rare sites of integration could no longer be distinguished from high frequency positions.
To overcome this limitation in sequencing, we developed a technology that measures the number of independent integration events that occur at single nucleotide positions. The technology, termed the serial number system, is based on randomizing eight base pairs in the tip of the Tf1 LTR. Each independent integration event is tagged with the "serial number" of the individual Tf1 element that was inserted. The serial numbers were introduced in a library of 250,000 clones of the Tf1-neo expression plasmid. The eight base-pair serial number can record as many as 65,000 independent insertions at each nucleotide of the S. pombe genome. Our first application of the technique detected 1.0 million independent insertions in diploid cells distributed among 130,000 positions (Reference 1). The integration numbers at individual positions varied over two orders of magnitude. Linear regression of independent replicas showed that we obtained a highly reproducible measure of integration in the intergenic sequences. The serial number data show the wide range of integration that occurred at individual nucleotides. The data confirmed the strong preference for promoter sequences and the levels of bias that favored specific promoters, including stress-response promoters. The advantage of the serial number data is that the quantitative measures of individual sites allowed us to study what distinguishes sites with high numbers of integrations.
One way to understand what features account for the high numbers of integration at specific chromosomal positions is to compare the nucleotide frequencies flanking positions with high numbers of integration to those with little integration. Logo analysis of the 150 positions with the highest numbers of integrations showed markedly higher nucleotide specificity with bit scores that were five times higher than the nucleotide composition flanking insertion sites with average numbers of integrations. This led us to ask how much influence insertion site sequence had on the genome-wide profile of integration. By ranking all integration events by the frequency of their repeated insertion, we found that sequence preference contributed to the efficiency of integration for 75% of the events. Importantly, we found that the 75% of the integration events occurred at just 33% of the total positions. Thus, the bulk of integration activity occurred at sites with a sequence signature. The sequence signature at high frequency insertion positions is just one determinant of the integration process. As described in the next section the quantitative integration data show that Sap1 is another key feature of strong integration sites.
Single nucleotide specific targeting of the Tf1 retrotransposon promoted by the DNA-binding protein Sap1 of S. pombe
While the serial number system identified specific sequences that contributed to integration efficiency, sequence did not account for the selection of promoters. We had tested the transcription factors known to activate stress-response promoters and found they do not contribute to the efficiency or position of Tf1 integration. However, a recent study of Switch activating protein 1 (Sap1), an essential DNA–binding protein in S. pombe, showed that Sap1 binds to genomic positions where Tf1 integration occurs. In order to determine whether Sap1 plays a role in Tf1 retrotransposition, we studied S. pombe with the temperature-sensitive mutant sap1-1 (Reference 2). At permissive temperature Tf1, transposition is reduced ten-fold compared with wild-type sap1+, and the defect was not the result of decreases in levels of Tf1 proteins or cDNA. The data argue that Sap1 contributes to the integration of Tf1. A mutation that results in 10-fold less integration might be expected to cause off-target integration. However, serial number sequencing of integration in cells with the sap1-1 mutation showed position changes in just 10% of the integration events.
In another approach to determine whether Sap1 contributes to integration, we compared the integration data from the serial number system with previously published maps of Sap1 binding created with ChIP-seq. Analysis of the ChIP-seq data showed that 6.85% of the S. pombe genome was bound by Sap1. Importantly, we found that 73.4% of Tf1 insertions occurred within these Sap1–bound sequences (Reference 2). An example of this close association can be seen in a segment of chromosome 1 (Figure 1). Another important observation is that a strong correlation was observed between levels of integration in intragenic sequences and the amount of Sap1 bound (R2=0.98). If Sap1 were directly responsible for positioning Tf1 integration, we would expect integration to take place at specific nucleotide positions relative to the nucleotides bound by Sap1. Using the ChIP-Seq data, we were able to identify a Sap1–binding motif, which closely resembled previously published motifs. We used the FIMO program of the MEME Suite to perform genomic searches, which identified 5,013 locations that matched this motif. The alignment of all these motifs revealed that 82% of all integration events cluster within 1 kb of this motif. Importantly, 43% of all integrations occurred within 50 bp of the motif and they had two dominant positions: 9 bp upstream and 19 bp downstream of the motif. The clustering of inserts at the Sap1 motif would be expected to occur if Sap1 covers its binding site on the DNA and directs integration to either side of the protein. Thus far, we have been unable to detect a direct interaction between Sap1 and Tf1 integrase (IN) with pull-down assays. However, our two-hybrid assays detected a strong Sap1–IN interaction. The two-hybrid result together with the strong alignments of integration with Sap1 motif sequence and the reduction in integration in the sap1-1 mutant argue that Sap1 plays an important role in Tf1 integration.
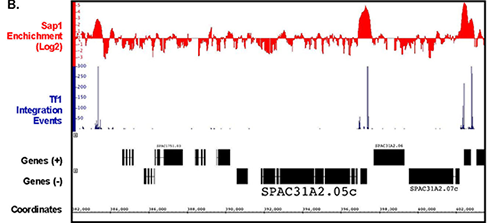
Click image to enlarge.
Figure 1. Serial Number integration data correlates with the position of Sap1 enrichment from ChIP-seq data.
A representative segment of chromosome 1 is shown.
A Long Terminal Repeat retrotransposon of Schizosaccharomyces japonicus integrates upstream of RNA pol III transcribed gene.
Transposable elements (TEs) are common constituents of centromeres. However, it is not known what causes this relationship. Schizosaccharomyces japonicus contains 10 families of Long Terminal Repeat (LTR)-retrotransposons, elements that cluster in centromeres and telomeres. In the related yeast, Schizosaccharomyces pombe, the LTR-retrotransposons Tf1 and Tf2 are distributed in the promoter regions of RNA pol II–transcribed genes. Sequence analysis of TEs indicates that Tj1 of S. japonicus is related to Tf1 and Tf2 and uses the same mechanism of self-primed reverse transcription. Thus, we wondered why these related retrotransposons localized in different regions of the genome.
To characterize the integration behavior of Tj1, we expressed it in S. pombe (Reference 3). We found Tj1 was active and capable of generating de novo integration in the chromosomes of S. pombe. The expression of Tj1 is similar to Type C retroviruses in that a stop codon at the end of Gag must be present for efficient integration. Seventeen inserts were sequenced, thirteen occurred within 12 bp upstream of tRNA genes and three occurred at other RNA pol III–transcribed genes. The link between Tj1 integration and RNA pol III transcription is reminiscent of Ty3, an LTR-retrotransposon of Saccharomyces cerevisiae, which interacts with TFIIIB and integrates upstream of tRNA genes. The integration of Tj1 upstream of tRNA genes and the centromeric clustering of tRNA genes in S. japonicus demonstrate that the clustering of this TE in centromere sequences is the result of a unique pattern of integration (Reference 3).
Retrotransposon Tf1 induces genetic adaptation to environmental stress.
Schizosaccharomyces pombe possesses a compact genome that tightly restricts retrotransposon expression under normal growth conditions. However, when retrotransposon Tf1 is expressed, it integrates into promoters of RNA Pol II–transcribed genes and, in many cases, this increases transcription of adjacent genes. The result, together with the Tf1 preference for stress-response promoters, led to the idea that Tf1 could be beneficial to its host by creating a pool of new alleles necessary for the host to survive changing environmental conditions. We tested the hypothesis by studying the Tf1 response to a stress such as exposure to cobalt and studying the fitness of cells with genomic insertions of Tf1 when exposed to cobalt.
Diverse cultures containing Tf1 integrated at 39,500 positions were grown competitively in cobalt. Cells with Tf1 at 141 positions greatly increased in proportion suggesting that the integrations improved growth in cobalt. Analysis of the positions and reconstruction of strains with single insertions indicate that Tf1 integration improved growth in cobalt by inducing key regulators of the TOR pathway. The results provide strong evidence that retrotransposons have the potential to promote evolution, and they identified mechanisms that mitigate the toxicity of cobalt.
Integration profiling: a whole-genome analysis of sequence function
The existing genome-wide methods for testing gene function consist largely of microarray hybridization and deep sequencing of RNA, techniques that infer function from patterns of gene expression. Despite the valuable information produced by these methods, they do not provide a direct demonstration of gene function. To address this need, we developed integration profiling, a simple method capable of directly probing the function of the single-copy sequences throughout the genome of a haploid eukaryote. With transposons that readily disrupt ORFs (open reading frames) and sequencing technology that can position over 250 million insertions per reaction, the analysis of a single culture can identify which sequences in a eukaryotic genome are functional. In previous work, we found that the 'cut and paste' DNA transposon Hermes from the housefly is highly active in S. pombe. The high rate of integration and the disruption of ORFs mean that Hermes is suitable for mutagenesis studies. With integration profiling, large populations of cells with transposon insertions are grown for many generations, depleting the culture of cells that have insertions in genes important for division. In one experiment, we passaged cells for 74 generations until 13.4% of the cells in the final culture contained an integrated copy of Hermes. We determined the positions of the insertions in the culture by ligation-mediated PCR followed by Illumina sequencing. We identified 360,000 unique insertion events that produced an average of one insertion for every 29 bp of the S. pombe genome (Reference 4). A survey of known essential genes revealed very few insertions per ORF, whereas neighboring nonessential gene ORFs had high numbers of insertions. Recently, a consortium systematically deleted the ORFs of S. pombe in heterozygous diploids and, after sporulation, designated which ORFs were essential (Kim et al., Nat Biotechnol 2010;28:617). Using these designations, we plotted the distribution of integration densities separately for the nonessential and essential ORFs. We also graphed the integration densities of a subclass of nonessential genes that, when deleted, resulted in small colonies. Clearly, the essential ORFs had significantly fewer insertions/kb than the nonessential ORFs, indicating that the integration profiles did indeed discriminate between essential and nonessential ORFs (Reference 4). Importantly, the nonessential ORFs required for full colony growth had intermediate densities of integration, indicating that intermediate levels of integration may be used to identify nonessential genes that nevertheless contribute to growth. The principal discrepancy between the designations made by the consortium and the Hermes integration is the group of 200 ORFs designated as nonessential, which exhibited very low levels of integration. Using PCR and DNA blotting, we found that the majority of these consortium designations were incorrect because the genes had not been successfully deleted. The results validate integration profiling as an accurate method for measuring gene function (Reference 4).
We extended the use of integration profiling to identify genes important for the formation of heterochromatin. Our initial strain contained a copy of ura4 (gene encoding orotidine monophosphate decarboxylase) within the centromeric sequence. The heterochromatin present in the centromeric sequence silenced the expression of ura4 and, as a result, allowed cells to grow in the presence of 5-fluorooritic acid (FOA). We then induced Hermes transposition and passaged cultures for many generations. Disruption of genes required for heterochromatin allowed ura4 to be expressed and, as a result, inhibited growth in a medium containing FOA. To identify the positions that tolerated disruption, we sequenced the integration sites of cells in the final culture. Our data set of one million integration positions contained, on average, one insertion for every 8 bp of the genome. We found that approximately 200 genes contained significantly fewer insertions than the remainder of the genome. Importantly, this gene set contained the majority of genes previously shown to contribute to heterochromatin formation. To test directly their contribution to heterochromatin and to characterize their mode of action, we are now analyzing candidates identified by integration profiling that have not previously been studied.
LEDGF/p75 interacts with mRNA splicing factors and targets HIV-1 integration to highly spliced gene.
The promise of immunotherapy of cancer and treatment of other diseases with gene therapy relies on retroviral vectors to stably integrate the corrective/therapeutic sequences in the genomes of the patient’s cells. First-generation gene therapy used vectors derived from gamma retroviruses that were successful in correcting X-linked severe combined immunodeficiency (SCID-X1). However, the integration pattern had a bias for promoter sequences that resulted in the activation of proto-oncogenes and progression to T-cell leukemia. Such adverse outcomes led to the use of lentivirus vectors for recent gene-therapy treatments. This switch to HIV-1–based vectors has occurred despite a fundamental lack of information about integration levels at specific genes, including proto-oncogenes. Structural and biochemical data show that HIV-1 IN interacts with the host factor LEDGF/p75 (a chomatin-binding protein and transcription coactivator), and the interaction favors integration in the actively transcribed portions of genes (transcription units). However, little is known about how LEDGF/p75 recognizes transcribed sequences and whether cancer genes are favored.
To measure integration levels in individual transcription units and to identify the determinants of integration-site selection, we generated a high-density map of the integration sites of a single-round HIV-1 vector in HEK293T cells (Reference 5). Improvements in sequencing methods allowed us to map 961,274 independent integration sites; most of the sites occurred in just 2,000 transcription units. Importantly, the 1,000 transcription units with the highest numbers of integration sites were highly enriched for cancer-associated genes, which raised concerns about the safety of using lentivirus vectors in gene therapy. Analysis of the integration site densities in transcription units (integration sites per kb) revealed a striking bias that favored transcription units that produced multiple spliced mRNAs and with transcription units that contain high numbers of introns (Figures 2A and 2B) (Reference 5). The correlations were independent of transcription levels, size of transcription units, and length of the introns. Analysis of previously published HIV-1 integration site data showed that integration density in transcription units in mouse embryonic fibroblasts also correlated strongly with intron number and that the correlation was absent from cells lacking LEDGF (Figures 2C and 2D). The data suggest that LEDGF/p75 not only tethers HIV-1 integrase to chromatin of active transcription units but also interacts with mRNA splicing factors. To test this, our collaborators used tandem MS to identify cellular proteins from nuclear extracts of HEK293T cells that interacted with GST-LEDGF/p75. The proteomic experiments found that LEDGF/p75 interacted with many components of the splicing machinery, including the small nuclear ribonucleic proteins (snRNP) SF3B1, SF3B2, and SF3B3 of U2 (a small nuclear RNA component of the spliceosome), U2–associated proteins PRPF8 and U2SURP, a factor of the U5 snRNP (SNRNP200), and many hnRNPs (heterologous ribonucleoproteins) that are associated with alternative splicing. The broad range of interactions with splicing factors suggested that LEDGF/p75 might contribute to splicing reactions. To test this, we performed RNAseq on HEK293T cells that were altered with TALEN endonucleases to truncate or delete the gene for LEDGF/p75, PSIP1. Analysis of the 11,000 transcription units that produced two or more spliced mRNA products showed that bi-allelic deletion of LEDGF/p75 significantly changed the ratio of spliced products of 4,305 transcription units (Reference 5). The results, together with our finding that integration in highly spliced transcription units was dependent on LEDGF, provide strong support for a model in which LEDGF/p75 interacts with splicing machinery and directs integration to highly spliced transcription units.
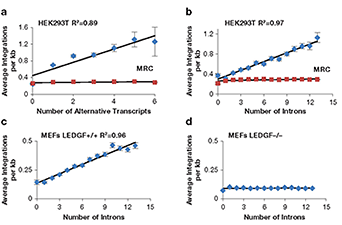
Click image to enlarge.
Figure 2. Integration density in transcription units correlates with amounts of splicing.
The numbers of HIV-1 integrations per kb in transcription units correlates with the amount of splicing (A and B). The preference for highly spliced transcription units depends on LEDGF (C and D).
Additional Funding
- NIH Intramural AIDS Targeted Antiviral Program (2015 and 2016)
Publications
- Chatterjee AG, Esnault C, Guo Y, Hung S, McQueen PG, Levin HL. Serial number tagging reveals a prominent sequence preference of retrotransposon integration. Nucleic Acids Res 2014; 42:8449-8460.
- Hickey A, Esnault C, Majumdar A, Chatterjee A, Iben J, McQueen P, Yang A, Mizuguchi T, Grewal S, Levin HL. Single nucleotide specific targeting of the Tf1 retrotransposon promoted by the DNA-binding protein Sap1 of Schizosaccharomyces pombe. Genetics 2015; 201(3):905-24.
- Guo Y, Singh P, Levin HL. A long terminal repeat retrotransposon of Schizosaccharomyces japonicus integrates upstream of RNA pol III transcribed genes. Mob DNA 2015; 6:19.
- Guo Y, Park JM, Cui B, Humes E, Gangadharan S, Hung S, Fitzgerald PC, Hoe KL, Grewal SI, Craig NL, Levin HL. Integration profiling of gene function with dense maps of transposon integration. Genetics 2013; 195:599-609.
- Singh P, Plumb M, Ferris A, Iben J, Wu X, Fadel H, Poeschla E, Hughes S, Kvaratskhelia M, Levin HL. LEDGF/p75 interacts with mRNA splicing factors and targets HIV-1 integration to highly spliced genes. Genes Dev 2015; 29:12.
Collaborators
- Nancy Craig, PhD, The Johns Hopkins Medical School, Baltimore, MD
- Shiv Grewel, PhD, Laboratory of Biochemistry and Molecular Biology, NCI, Bethesda, MD
- Stephen Hughes, PhD, Retroviral Replication Laboratory, HIV Drug Resistance Program, NCI, Frederick, MD
- Mamuka Kvaratskhelia, PhD, Ohio State University, Columbus, OH
- Philip McQueen, PhD, Mathematical and Statistical Computing Laboratory, CIT, NIH, Bethesda, MD
- Eric M. Poeschla, MD, University of Colorado, Aurora, CO
Contact
For more information, email henry_levin@nih.gov or visit http://sete.nichd.nih.gov.